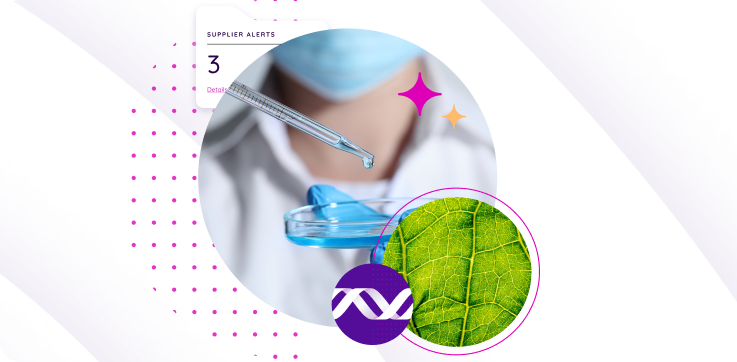
In our previous posts, we discussed the evolution of quality management systems (QMS) and AI tools and technologies that are accelerating quality in the life sciences industry.
In this third and final installment, we take a look forward with Harsh Thakkar, Qualtivate CEO, to explore future trends in AI within the life sciences and challenges that will need to be addressed.
Speculating on Future Trends in AI
As AI continues to evolve, its impact on the life sciences industry will expand in several key areas.
Emerging Technologies
Emerging AI technologies are set to further advance the life sciences industry. Some exciting trends include quantum computers that could process complex biological data much faster than current systems, enabling breakthroughs in drug discovery and personalized medicine. Another trend, federated learning,allows AI models to learn from data across multiple locations without centralizing it, enhancing data privacy and security and enabling predictive maintenance and better quality control. Additionally, advanced AI algorithms will identify new biomarkers for diseases, leading to more accurate diagnostics and targeted therapies.
Personalized Medicine
AI will play a crucial role in the advancement of personalized medicine. By analyzing genetic, environmental, and lifestyle data, AI can help create individualized treatment plans. This tailored approach will improve patient outcomes and reduce negative effects.
AI in Clinical Trials
AI will continue to transform clinical trials by improving patient recruitment and study designs, and ensuring real-time monitoring. This will lead to faster, more efficient trials with higher success rates.
AI-Powered Drug Discovery
The future of drug discovery lies in AI’s ability to predict molecular behavior and identify promising compounds. AI-driven platforms will significantly shorten the time and cost associated with bringing new drugs to market.
Remote and Decentralized Trials
AI will facilitate the shift towards remote and decentralized clinical trials. Virtual trials, enabled by AI-powered monitoring tools, will make participation easier for patients and increase the diversity and size of trial populations.
Overcoming the Top Challenges
The AI revolution is not without its challenges. Below are the key challenges organizations are faced with:
Data Privacy and Securit
AI systems handle huge amounts of sensitive data, which makes ensuring privacy and security is critical. Organizations will have to develop frameworks to protect patient information and comply with regulations.
Regulatory Compliance
The regulatory landscape for AI in life sciences is still evolving. Ensuring that AI systems meet regulatory requirements and standards will be a top challenge. Organizations will need to work closely with regulatory bodies to navigate this complex environment. Compliance is crucial because, as Thakkar states, “regulatory compliance is more than adhering to rules; it’s about ensuring that every part of your operations upholds the highest standards.”
Ethical Considerations
AI systems must be designed and deployed ethically. Issues such as bias in AI algorithms, transparency in decision-making, and the potential for job displacement need to be addressed. Establishing ethical guidelines and practices will be essential for organizations in this new landscape.
Integration with Existing Systems
Integrating AI technologies with existing systems and workflows can be challenging. Ensuring seamless interoperability and minimizing disruption to current operations will require careful planning and execution.
Skills and Talent Shortage
The demand for AI expertise in life sciences is growing rapidly. Organizations may face a shortage of skilled professionals who can develop, implement, and manage AI systems. Investing in training and education will be essential to building a capable workforce.
Cost of Implementation
The initial cost of implementing AI technologies can be high. Organizations will need to justify the investment by demonstrating the long-term benefits and return on investment. Finding cost-effective solutions and managing budgets will be a key challenge.
What’s Next for AI in Life Sciences?
There’s much more to come on the AI front. The future of AI in life sciences has great potential, with emerging technologies poised to accelerate innovation across the industry.
Thakkar says, “AI is going to help us become more proactive, preventing issues from happening by understanding all the data. If we’re going to be using AI in quality management it’s obvious that we need to have good data integrity and data governance and good data management habits. Without that we’re just wasting money on AI because AI won’t work—then we’ll blame the tool and not our habits. Good data integrity practices help build quality into our processes and systems.”
Evolving technology will continue to transform processes, and improve efficiencies and patient outcomes across the industry. The ongoing development and application of AI will play a crucial role in driving these benefits.
We’ve come to the end of our series but we’re still here to answer any questions you may have about AI-powered eQMS solutions. Reach out to request a demo today.